Protecting the Data that Feeds your AI Strategy
- Date: Jul 18, 2023
- Read time: 10 minutes
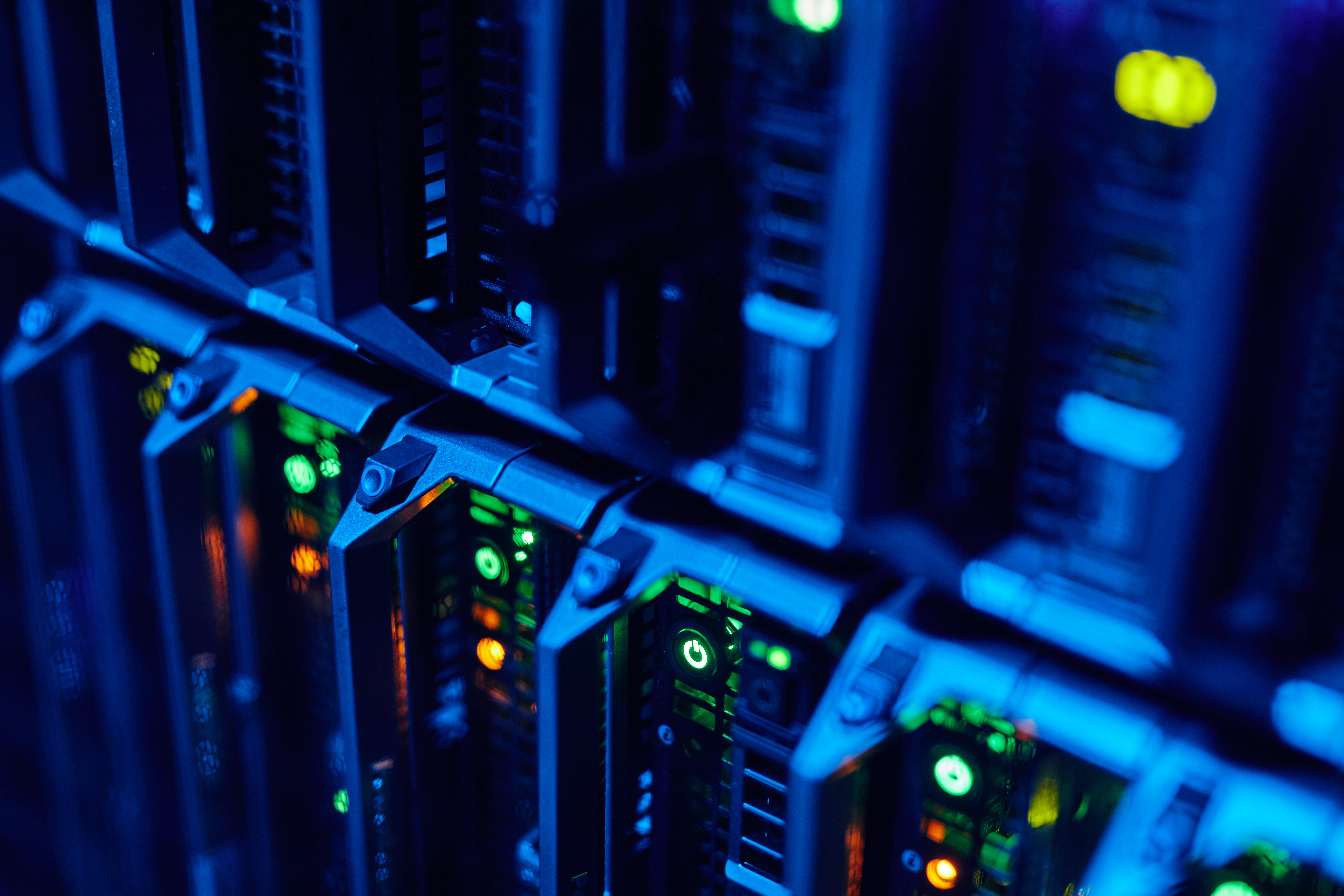
Why prevention is the new recovery
Generative AI: You’re hearing about it everywhere now, but what exactly is it? Generative AI refers to a branch of artificial intelligence that’s focused on creating or generating new content – everything from images, text, to music and even video – based on patterns and examples extrapolated from seed data used to “train” the model. It uses deep learning models and networks to identify underlying patterns in data and generate new content with characteristics that are similar to the training examples. In generative AI, training data refers to the dataset used to train a generative model. It’s basically a collection of examples or samples from which the model extrapolates and captures patterns, relationships, and statistical properties of the data.
Our security presence at the data layer is the “red line” of defense, detecting (and thwarting) what – if left unchecked – could become worse… much worse.
Generative AI has the potential to revolutionize many industries by enabling the creation of new and highly-personalized content, assisting in complex tasks, and – arguably – enhancing creativity. Of course, ethical considerations, data privacy, and potential biases in generated content need to be carefully addressed to help ensure that this exciting new technology is being used responsibly and beneficially.
Data types used in training
The type of data used to train a model will depend on the task at hand. For example:
- Image Generation: In image generation tasks, the training data typically consists of a large collection of images. These images can be from multiple sources, including publicly available image databases or custom datasets created for a specific use case. Ideally, the images in the training data should be representative of the desired output, but can represent a diverse range of styles, subjects, and variations.
- Text Generation: For text generation, you might start with a collection of text documents or sequences. This could be everything from books and articles to web pages, or any other textual content. The goal is to provide the model with a rich source of language patterns, grammar, and semantic relationships.
- Music Composition: Training data might include musical scores, audio recordings, even MIDI files. Taken together, these will enable the model to identify and “learn” musical patterns, harmonies, melodies, and rhythms, enabling it to generate unique new musical compositions.
- Video Generation: This would entail ingestion of video data that might include collections of videos from various sources or even custom-created datasets. The videos provide the generative model with visual information and temporal patterns necessary to generate new video content.
- Simulation and Augmentation: Generative models can learn to simulate and augment data that can be used for training other AI models. By generating synthetic data, it can help improve the performance and generalization the algorithms used for machine learning.
When assembling training data, it’s critical that you curate and prepare the data carefully to ensure that you’ve got quality, diversity, and a practical representation of what you’re looking for in your desired output. Preprocessing of the training data is frequently required – cleansing data, normalizing formats, or augmenting the dataset – to enhance the model’s ability to
generalize and generate high-quality output. Regardless, both the training data and the generated output will ultimately take the form of unstructured data… and a lot of it! And the unique nature of unstructured data requires a thoughtful approach to protecting, managing, and storing it.
An increased data footprint means a larger attack surface
Generative AI models require massive amounts of input data and generate equally massive amounts of output data. Of course, this requires the ability to efficiently – and cost-effectively – manage, store, and protect all that data. Having an increased data footprint creates a larger attack surface that’s quickly becoming attractive to cybercriminals, and even adds new challenges, such as obtaining and managing consent where appropriate, and ensuring compliance with relevant data protection regulations in the regions in which you do business. Some additional considerations for your data protection strategy include:
- Data Minimization: While Generative AI relies on diverse and representative datasets, you need to balance the need for data diversity against the principle of data minimization. You’ll want to carefully evaluate the value of collecting and storing certain types of data in order to minimize potential risks and liabilities that might occur with a data breach or unauthorized access, both external and internal.
- Data Anonymization and De-identification: You may need to anonymize or de-identify data in order to protect individual privacy. Having a thoughtful anonymization strategy – such as removing personally identifiable information (PII) or applying differential privacy methods – can help mitigate privacy risks.
- Data Security: As your data footprint increases, so too does the importance of having in place robust data security measures. You’ll want to implement strong security protocols to protect the data used for training generative AI models, to help ensure that it’s safeguarded against unauthorized access, breaches, or misuse. This might include encryption, controlled access, regular security audits, and employee training on best practices for data security.
- Ethical Use of Generated Content: Because Generative AI can generate content that mimics or imitates human-generated content, you need to consider the ethical implications of the generated content. This might potential misinformation, biases, or its use in the creation of deepfake images, video, and audio. Your data protection strategy will need to be updated to ensure that your generated content is being used ethically and responsibly.
- Regulatory Compliance: You’ll also need to ensure that your use of Generative AI complies with applicable data protection regulations, such as the European Union’s General Data Protection Regulation (GDPR). This includes adhering to principles of lawful processing, transparency, purpose limitation, and data subject rights. You’ll also want to consider conducting Data Protection Impact Assessments (DPIAs) to assess and mitigate any potential risks associated with generative AI.
With great data comes great responsibility
Overall, Generative AI introduces new considerations for your data protection strategies. You’ll need to carefully evaluate and adapt your data handling practices to address new challenges that come with Generative AI, while at the same time maintaining compliance with relevant data protection regulations, as well as ensuring the privacy and security of that data.
As your data footprint increases, you’ll need to reevaluate your infrastructure needs, specifically around storage and processing. Managing and maintaining the infrastructure for storing and processing such large datasets can be costly and resource-intensive. For example, where your data currently resides may not be the most cost-efficient location or tier. And where your data is processed is typically nowhere near where it actually resides… with an impact on ingress and egress charges.
What’s more, properly securing unstructured data has become critical, because now you’ve got terabytes – maybe even petabytes – of vital data that’s potentially exposed to various flavors of ransomware, exfiltration, untrusted access, and more.
Traditional backup is no longer enough
In an IT organization, we all know that backup is table stakes. It’s not unusual for an organization to have multiple backup solutions, spanning different lines of business or different functions. Depending on the regulatory environment in which you’re operating, you’ll need to make a copy of your data (sometimes even an additional air-gapped copy); you’ll need a backup copy; and you’ll need to be able to easily recover from that backup or air-gapped copy.
But backup alone simply isn’t enough for a rapidly expanding data footprint. And whether your data growth is organic or is a byproduct of analytics or monetization, it’s become critical to be able to identify which data should go somewhere else… for operational efficiency, for cost savings… whatever. And that’s the point at which you need to shift your data strategy from backup and copies to Intelligent Orchestration. You need to be able to understand exactly who is touching which data, and when. By understanding those aspects, you can plot-out how, when, and where you move your data… whether it’s to lower-cost on-prem options, or inexpensive, deep archive cloud tiers. And with Intelligent Orchestration, you can simplify and even automate these processes. Imagine being able to take a multi-petabyte pool of data and, with full orchestration control, making a copy of it for recovery or compliance purposes; or segmenting it based on access patterns, frequency and purpose. Simplifying these processes can help you increase operational efficiency, reduce cost, aid compliance, and even set yourself up to monetize your data!
In today’s active cyberthreat climate, you need to be able to detect when someone – internal or external – is accessing your data. This is critical not only for audit purposes, but also because access can often be the first indication of trouble. Unusual data access patterns are often indicators of the initial stages of ransomware or exfiltration. As files are being encrypted, it creates a recognizable pattern. Superna software detects hundreds of unusual patterns, and our detection catalog is constantly being refreshed. We can detect when a burrowing event or activity suggestive of ransomware is taking place. Upon detecting an anomaly, we can even trigger an air-gap of data and lock-out the IP address that’s posing the threat. And because we’re doing this at the data layer, it really is next-level security.
What if the “bad actor” is someone who already has access to your network? Superna has an AI learning layer that’s constantly looking at data access patterns, allowing us to detect when someone operating at the data layer is behaving in a way that’s unexpected. Maybe someone who normally accesses certain data environments is now touching several other shares, perhaps copying or deleting data. Upon detection, we can freeze their access and lock them out entirely, even trigger action via a SOAR security automation framework. Our security presence at the data layer is the “red line” of defense, detecting (and thwarting) what – if left unchecked – could become worse… much worse.
Superna closes the cybersecurity gap
Superna helps close the cybersecurity gap. It looks easy – but auditing, securing, and moving complex, unstructured data at petabyte scale is challenging, and it’s where we’ve spent nearly 15 years of engineering to perfect it! Here’s how we do it:
- Intelligent Orchestration helps simplify ongoing management of what can be really challenging on an enormous data footprint.
- We provide support for forensics and compliance: “Who touched what, when, and where?” What we’re looking at is to determine who is accessing your data, and what – if anything – is actually happening to that data.
- Finally, we apply the NIST cyber security framework to the largest and most complex data layer on the planet: Unstructured Data.
Through our market-tested technology and years of experience in managing unstructured data, we provide our customers with greater insight and control. And by running security at the data layer, we’re able to bolster security for these large datasets, regardless of where they reside: On-prem, in the cloud, and in hybrid, multi-cloud environments.
Lear more
Want to learn more? Contact us to speak with a data protection expert or to schedule a demo.
Featured Resources
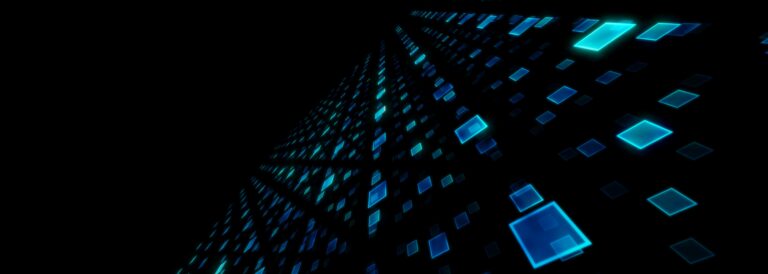
Mastering Cybersecurity Insurance Negotiations: A Comprehensive Guide
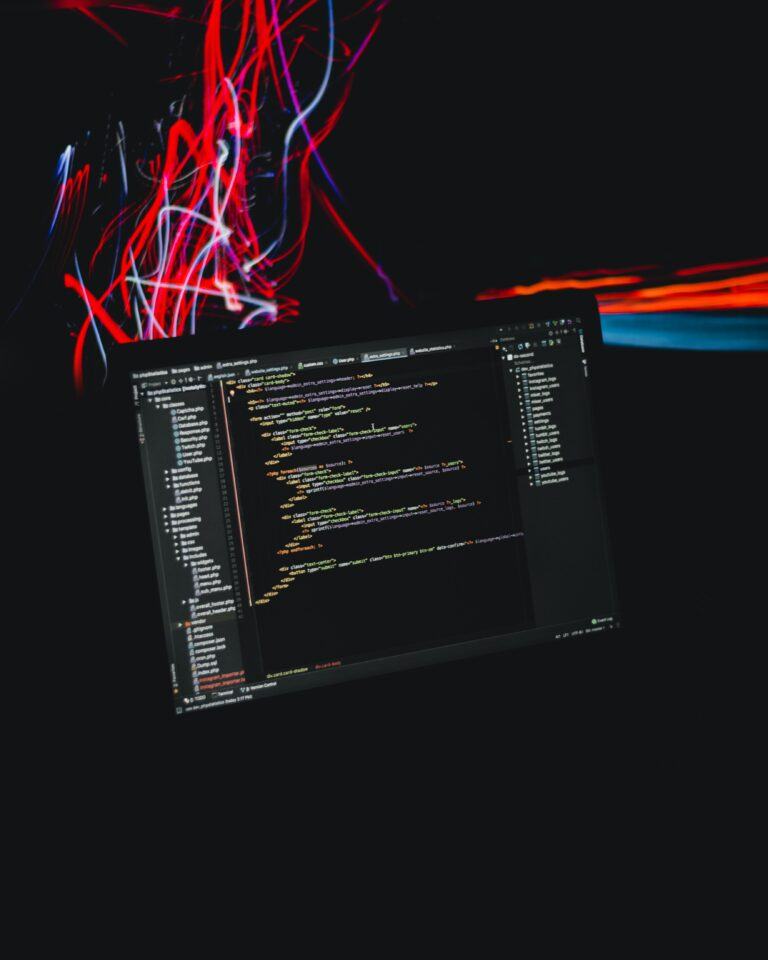
Navigating the Digital Menace: A Beginner’s Guide to Ransomware
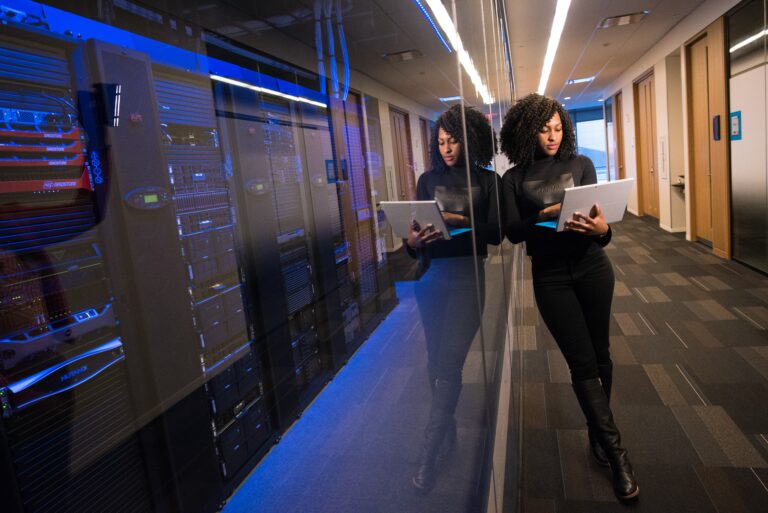