How can Cyberstorage increase your probability of detecting an attacker before the attack?
- Date: Jan 29, 2025
- Read time: 5 minutes
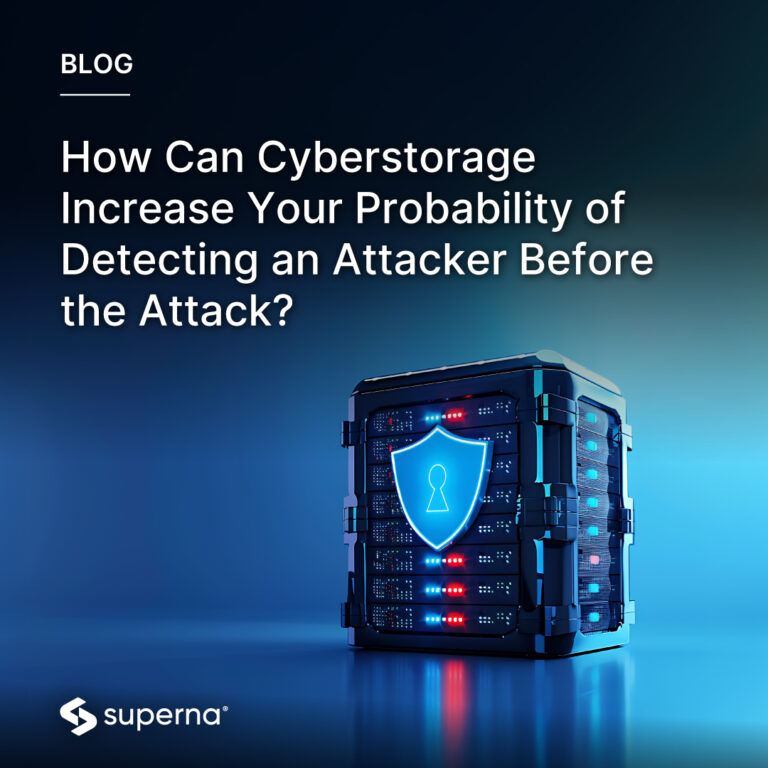
About the Author
Andrew MacKay – Chief Technology & Chief Strategy Officer Of Superna
This blog builds on the article Compound Probability: You Don’t Need 100% Coverage to Win (a great read) , which demonstrates how incremental improvements in detection coverage can disproportionately enhance your defense against attackers. By integrating data layer detection, such as Superna Cyberstorage, you can use the attacker’s ultimate objective—accessing data—against them.
This blog post expands on the compound probability model discussed in the previous article, demonstrating how Cyberstorage can increase your attack surface coverage, significantly reduce an attacker’s success rate, and improve your overall security posture through targeted enhancements in detection coverage.
This blog will explain why Cyberstorage is the only technology that can significantly increase the chances of early Cyber attack detection and give you a strategic advantage in protecting your data.
Leveraging the Attacker’s Objective Against Them
Attackers ultimately aim to access data, whether to exfiltrate, encrypt, or manipulate it. Each step of their attack, including persistence, lateral movement, and privilege escalation, serves this singular goal. By understanding their techniques and objectives, defenders can focus on strategically deploying detection capabilities that exploit the attacker’s need to continually assess their access to data.
The results of the analysis in this blog emphasizes that detection engineers should prioritize high-impact detection points that maximize attack surface coverage. Instead of focusing on edge cases, targeting behaviors tied directly to data access provides consistent opportunities to detect and mitigate attacks.
Actions Attackers Take During an Attack
Beyond persistence, lateral movement, and privilege escalation, attackers engage in various other actions. Many of these steps tie directly to opportunities for detection, especially at the data storage layer:
- Initial Access:
- Gaining a foothold through phishing, exploiting vulnerabilities, or using stolen credentials.
- Discovery Targets: Scanning the network to identify hosts, services, configurations, and vulnerabilities.
- Data Discovery Targets (Data Attack Detection):
- Enumerating file shares, Active Directory, or installed software to locate sensitive data.
- Command and Control (C2):
- Establishing communication with a remote server to exfiltrate data, issue commands, or maintain control.
- Defense Evasion:
- Disabling security tools, clearing logs, or employing fileless malware to avoid detection.
- Credential Access:
- Harvesting account credentials from memory, keyloggers, or password files.
- Data Exfiltration (Data Attack Detection):
- Extracting sensitive data to external locations.
- Execution (Data Attack Detection):
- Ransomware attack on the data
- Running malicious code to disrupt or encrypt data.
- Ransomware attack on the data
- Data Manipulation (Data Attack Detection):
- Modifying or encrypting critical data as part of sabotage.
- Resource Hijacking:
- Using compromised systems for unauthorized purposes such as cryptocurrency mining.
- Impact (Data Attack Detection):
- Delivering the intended payload, such as disrupting services, encrypting data, or manipulating critical systems data.
These stages are often part of an attacker’s strategy based on frameworks like MITRE ATT&CK. Each of these stages presents opportunities for detection, particularly if attackers are interacting with centrally managed data storage systems like NAS devices. By focusing detection efforts on these critical touchpoints, defenders can significantly increase their chances of early detection.
Detection Logic Behind the Graph
The accompanying graph visualizes the probability of detecting an attacker across a 5-step attack sequence. An attack sequence consists of major steps to breach data, where at each step the attacker checks if they have access to data of interest.
Below are the detailed assumptions and notes for each scenario:
- Referenced Article Example with 25% Coverage:
- Assumes the attacker has 4 possible actions per step, with 25% detection coverage for one of these actions.
- Assumption:
- Detection rate is 100% successful if the attacker chooses a path with a detection capability.
- No Storage layer early detection capability exists
- Detection probability compounds with each step, reaching ~76% success rate by step 5.
- Superna AI Early Detection Combined with existing 25% Attack vector Coverage:
- At each step of the attack the attacker validates data access to see if the step gained access to data for compromise (data exfiltration, destruction, manipulation, Ransomware attack). After each major step in the attack sequence the data inspection step is executed by the attacker.
- This model combines the two approaches and computes the probability of successful early detection when early Cyberstorage detection is used.
- Assumption:
- Assumes initial NAS detection rate starts at 85% and improves by 10% per step as the AI learns from more data. AI models get more accurate detection with more data points.
- The AI’s learning curve improves detection speed as more data is available resulting in accelerated early detection probability.
- Detection Probability by step 5, approaches ~99.999%, showcasing the power of adaptive learning systems at the storage layer combined with existing endpoint, network detection domains.
Key Insights from the Analysis
- Compounding Effect: Detection probabilities increase exponentially as the attack progresses, even with partial coverage at each step but accelerated early detection is only possible because the attacker checks data access at each step.
- Guaranteed Detection Vector per step: Scenarios leveraging Superna’s AI early detection Cyberstorage benefit from the attacker’s repeated interaction with NAS storage, significantly improving detection odds.
- Layered Defense: Combining multiple detection methods provides the highest probabilities of success, emphasizing the importance of comprehensive, multi-layered security strategies.
Conclusion
Superna Cyberstorage accelerates early detection rates by up to 70%, achieving over 98% detection success probability. By adding data-specific detection to your security stack, you enhance early threat identification while bolstering your ability to respond to and recover from attacks. The model also shows that storage domain detection combined with existing methods provides the fastest , most reliable method for detecting early signs of a breach.
Attackers inevitably target data. By leveraging this fact, Superna Cyberstorage turns the attacker’s objective into their Achilles’ heel. Its robust detection capabilities, coupled with machine learning-driven improvements, address every phase of a security incident—from reconnaissance to recovery—ensuring your data remains secure.
For details on how the probability was computed to show the effects of compound probability is below.